The role of machine learning in personalized education: a closer look
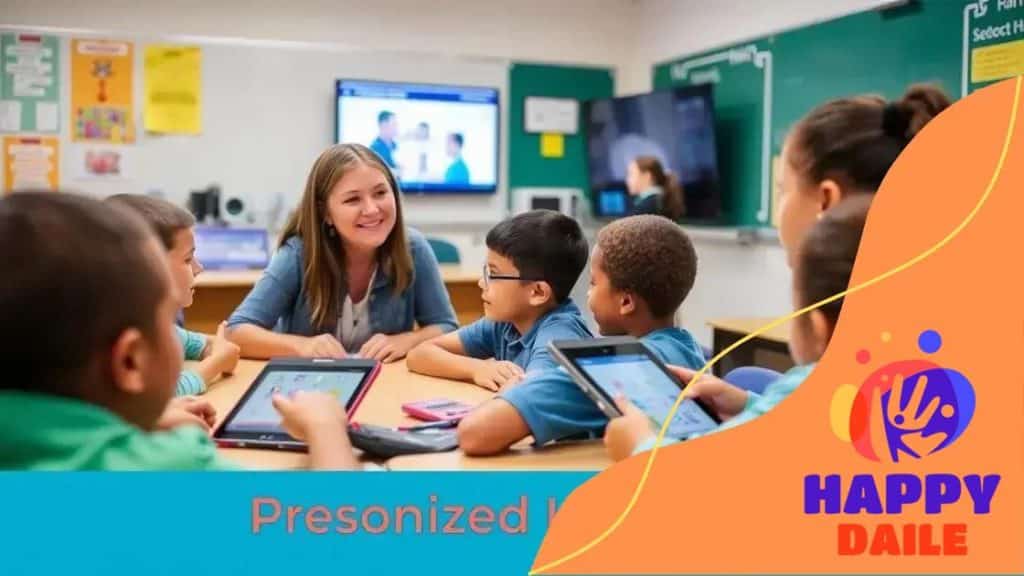
Anúncios
The role of machine learning in personalized education enhances learning by tailoring content to individual student needs, improving engagement, and providing data-driven insights for teachers.
The role of machine learning in personalized education is a game-changer for students and teachers alike. Imagine a learning environment that adapts to each student’s unique style and pace. Isn’t that exciting? Let’s delve into how this technology transforms the educational landscape.
Anúncios
Understanding machine learning and education
Understanding machine learning in education is essential for grasping how it can benefit both students and teachers. In this section, we will look at the basics of machine learning and its impact on learning environments.
What is machine learning?
Machine learning is a branch of artificial intelligence that allows systems to learn and improve from experience without being explicitly programmed. It involves developing algorithms that analyze data, identify patterns, and make decisions based on that information. This process is crucial in the context of education, as it enables personalized learning experiences.
Benefits of machine learning in education
Incorporating machine learning in education brings numerous advantages:
Anúncios
- Personalized learning: Machine learning tailors educational content to meet individual student needs.
- Immediate feedback: Students receive timely feedback, helping them understand concepts better.
- Enhanced engagement: Interactive learning tools keep students motivated.
These benefits contribute to a more dynamic and effective learning experience.
Moreover, machine learning can help teachers by providing data-driven insights into student performance. Educators can easily identify areas where students struggle and adjust their teaching methods accordingly. This proactive approach creates a more supportive learning environment.
Examples of machine learning in education
There are many practical applications of machine learning that enhance education:
- Adaptive learning platforms: These platforms adjust content based on student performance.
- Automated grading systems: They save teachers time and ensure consistent assessment.
- Chatbots for tutoring: Chatbots provide students with instant assistance outside the classroom.
These examples showcase how machine learning integrates seamlessly into educational settings, improving overall effectiveness and student satisfaction.
Benefits of machine learning in personalized learning
The benefits of machine learning in personalized learning are profound and transformative. By leveraging data and algorithms, educators can tailor learning experiences to meet the needs of each student.
1. Individualized Learning Paths
Machine learning helps create individualized learning paths for students. These paths are designed based on students’ strengths and weaknesses. For example, if a student excels in math but struggles with reading, the program adjusts the difficulty and content types accordingly, ensuring optimal learning.
2. Data-Driven Insights
Educators gain valuable insights through data analysis. Machine learning identifies trends and learning patterns, allowing teachers to make informed decisions. This leads to better resource allocation, instructional strategies, and student support.
3. Enhanced Engagement
Engagement is crucial for effective learning. Machine learning provides interactive tools that make learning more engaging. Gamified elements and adaptive challenges keep students motivated and eager to learn.
4. Immediate Feedback
With machine learning, students receive immediate feedback on their performance. This feature helps them understand what they need to improve. Prompt feedback also fosters a growth mindset, pushing students to strive for better results without delay.
5. Scalability and Accessibility
Another major benefit is scalability. Machine learning systems can serve countless students simultaneously. Whether in a crowded classroom or an online platform, these systems ensure that all learners receive quality educational support.
In summary, machine learning opens doors to a more personalized and effective learning experience. By focusing on the individual needs of students, educators can foster a more enriching educational environment for everyone.
How machine learning customizes learning paths
Machine learning customizes learning paths in a way that adapts to individual student needs. This personalization makes education more effective and engaging for everyone involved.
Understanding Customization
At its core, machine learning analyzes vast amounts of data to identify patterns in student behavior and performance. By understanding these patterns, educational platforms can create tailored experiences. For example, if a student struggles with specific math problems, the system can offer additional resources and practice in that area.
Adaptive Learning Systems
Adaptive learning systems are one of the most significant applications of machine learning. These systems continuously adjust the content and difficulty based on real-time feedback. Students receive exercises that match their skill levels, ensuring they are neither bored nor overwhelmed.
- Personalized content: Each student gets learning materials that fit their unique needs.
- Real-time adjustments: The system modifies challenges based on student responses.
- Targeted support: Teachers receive insights to assist struggling students more effectively.
These features create a dynamic educational environment that fosters growth.
Benefits of Customized Learning Paths
One major benefit of customized learning paths is the increase in student motivation. When students engage with material that is interesting and relevant to them, they are more likely to stay focused and perform well. Additionally, by catering to different learning styles—such as visual, auditory, or kinesthetic—machine learning helps every student reach their full potential.
Examples in Action
Many educational platforms utilize machine learning to customize learning paths. For instance, programs like Khan Academy and DreamBox offer tailored lessons based on student progress. This customization ensures that each student’s journey is as effective as possible, allowing them to build on their strengths and address their weaknesses seamlessly.
Challenges and limitations of machine learning in education
While machine learning has numerous advantages in education, it also presents several challenges and limitations that schools must navigate. Understanding these issues is crucial for effective integration.
Data Quality and Availability
One significant challenge is the quality of data. Machine learning algorithms rely on high-quality data to function correctly. If the data is inaccurate or biased, the results will be too. For instance, if a school collects insufficient data on students’ needs, the personalized learning paths generated might not be beneficial.
Integration with Existing Systems
Another challenge is the integration of machine learning tools with existing educational systems. Schools may face difficulties merging new technologies with traditional teaching methods. This disconnect can lead to resistance from educators who are unfamiliar with machine learning applications.
Costs and Resources
Implementing machine learning solutions often requires significant investment in infrastructure and training. Many schools lack the necessary resources or funding to adopt sophisticated systems. This limitation can hinder their ability to benefit from the technology.
Privacy and Ethical Concerns
Privacy is a major concern as well. Collecting data on students involves ethical responsibilities. Schools must ensure that they protect students’ personal information while complying with laws such as GDPR and FERPA. These regulations can complicate data collection and usage strategies.
Dependence on Technology
Lastly, over-reliance on technology can be a limitation. While machine learning can enhance learning experiences, there’s a risk that educators may lean too heavily on automated systems. This might reduce human interaction, which is vital for building interpersonal skills in students.
By recognizing these challenges, educators and policymakers can take steps to mitigate the risks while maximizing the benefits of machine learning in education.
Real-world applications of machine learning in schools
Real-world applications of machine learning in schools are transforming education in exciting ways. These technologies help teachers improve the learning experience and support students in unique ways.
Personalized Learning Programs
One notable application is the use of personalized learning programs. These programs adapt to each student’s learning pace and style. For instance, platforms like DreamBox and Khan Academy use algorithms to analyze student performance, offering customized lessons that fit individual needs.
Predictive Analytics
Another powerful tool is predictive analytics. Schools can use machine learning to identify which students may need additional support. By analyzing past performance data, educators can proactively intervene before students fall behind.
- Identifying at-risk students: Early detection of potential struggles leads to timely interventions.
- Improving attendance: Insights help schools address factors affecting student attendance.
- Enhancing resource allocation: Data-driven decisions ensure resources are used effectively to support students.
This proactive approach not only helps students succeed but also empowers teachers to make informed decisions.
Automated Administrative Tasks
Machine learning also streamlines administrative tasks. Schools can automate processes like grading, attendance tracking, and scheduling. By reducing the workload on educators, they can focus more on teaching.
Additionally, chatbots powered by machine learning can handle common queries from students and parents, providing quick responses. This use of technology improves communication and efficiency within the school environment.
Enhanced Assessment Tools
Educational assessments are becoming more dynamic with the help of machine learning. Tools like Gradescope allow for faster and more consistent grading. These platforms provide insights into student comprehension, helping educators adjust their teaching methods accordingly.
Through these real-world applications, machine learning is not only enhancing educational experiences but also creating a more efficient school system. This technology supports personalized learning, improves administrative tasks, and equips educators with the tools they need to facilitate effective teaching.
FAQ – Frequently Asked Questions about Machine Learning in Education
How does machine learning personalize learning paths for students?
Machine learning analyzes student performance and adapts the educational content to fit individual learning styles and paces.
What are the main benefits of using machine learning in schools?
Machine learning enhances engagement, provides immediate feedback, and helps in identifying students who may need additional support.
What challenges do schools face when implementing machine learning tools?
Schools may encounter issues such as data quality, integration with existing systems, and costs associated with technology adoption.
Can machine learning systems automate administrative tasks?
Yes, machine learning can automate grading, attendance tracking, and other administrative processes, allowing teachers to focus more on teaching.